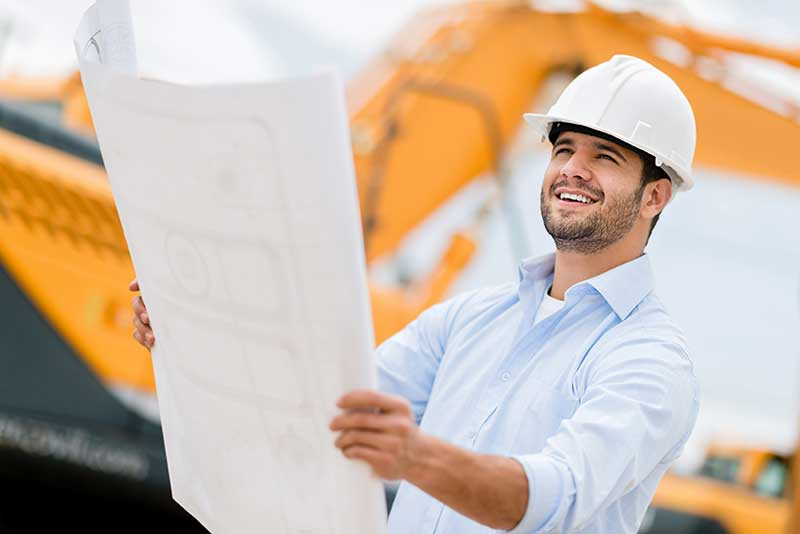
Cały rok marzymy o urlopie W sieci jesteśmy w stanie odnaleźć setki portali ogłoszeniowych, oferujących nam szansę zamieszczenia osobistego anonsu za niewielką opłatą a niektóre, całkowicie darmowe. Podzielone na kategorie tematyczne witryny są przejrzyste oraz łatwo odszukać na nich dobry dział, w jakim pragniemy się zareklamować bądź ogłosić, że poszukujemy pracy czy lokalu. W aktualnych czasach, kiedy papierowe gazety wypierane są przez serwisy informacyjne, takie portale cieszą się olbrzymią popularnością, głównie pośród młodych ludzi, studentów proponujących swoje usługi w temacie wiadomości kraków, wypatrujących pokoju czy dla pracodawców, chcących zatrudnić kogoś młodego. Portale ogłoszeniowe, te znane oraz te dopiero powstające gwarantują [ Read More ]